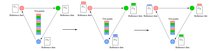
- Resources
- Related Research Areas
- Data Mining and Knowledge Discovery
Detecting anomalous behavior from terabytes of flight record data has emerged as a crucial component for many systems for Aircraft Health Management. Very often, however, flight record data collected from various aircrafts cannot be directly aggregated for anomaly analysis due to ownership and privacy issues. Our project is investigating a novel framework for anomaly detection from distributed data sources that cannot be directly merged. In the proposed approach, normal behavior is first learned from data on individual aircraft using any standard (single site) anomaly detection method, and then these models are combined when predicting anomalous behavior for a new aircraft. We have investigated several semi-supervised and unsupervised anomaly detection algorithms for learning normal behavior, as well as four methods for combining the proposed anomaly detectiion models. Our experiments, performed on simulated data, real data obtained from NASA's ASIAS project, and operational data from SIkorsky have shown that our proposed approach can achieve comparable, or even in some cases better, anomaly detection rates as compared to a model built from the dataset obtained by mergin all individual datasets.
Discussions
Popular Resources
-
Algorithms for Speeding up Distance-Based Outlier Detection
A Publication, Scalable Outlier Detection on Very Large Datasets - 12 years, 8 months ago
Shared By: Kanishka Bhaduri
The problem of distance-based outlier detection is difficult to solve efficiently in very large datasets because of potential quadratic time complexity. We address this problem ...
Related Projects
-
Wikis across NASA
2 members
-
Test project
1 members
-
Need help?
Visit our help center